

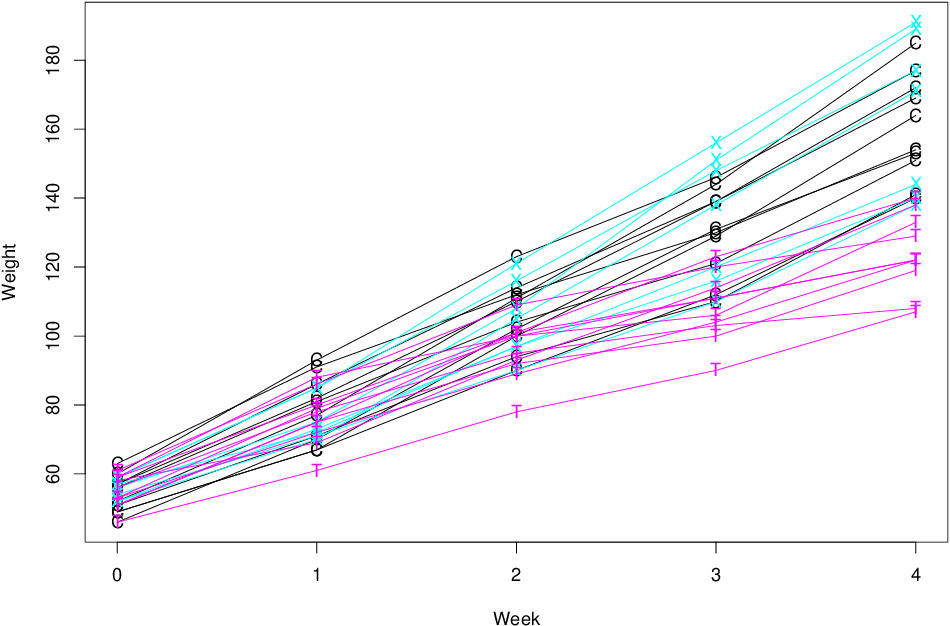
Moving average is itself considered as one of the most rudimentary methods of forecasting. We would first understand these components one by one. It is one of the most popular techniques used for time series analysis and forecasting purpose.ĪRIMA, as its full form indicates that it involves two components : It is also known as Box-Jenkins approach. Tutorial - Basics of Time Series ModelingĪRIMA stands for Auto-Regressive Integrated Moving Average. His annual salary can be considered a time series and it is clear that every year's salary is function of previous year's salary (here function is appraisal rating). Every years he is appraised and salary reached to a level of $20,000 per month in year 2014. X starts his job in year 2010 and his starting salary was $5,000 per month. Value at time "t" can depend on one or various lags of various order. It means value (V) at time "t" is a function of value at time "n" instance ago with an error (e). ARIMA technique exploits the auto-correlation (Correlation of observation with its lags) for forecasting. This assumption is the basis of performing a time series analysis. Typically, in a time series it is assumed that value at any given point of time is a result of its historical values.

daily Stock Exchange closing point, weekly sales and monthly profit of a company etc. This tutorial explains the theoretical concepts of time series and ARIMA modeling and how we can forecast series using ARIMA with R.Ī time series is a data series consisting of several values over a time interval.
